class: center, middle, inverse, title-slide # Drawing inference
🤔 --- layout: true <div class="my-footer"> <span> by Dr. Lucy D'Agostino McGowan </span> </div> --- ## <i class="fas fa-laptop"></i> `Porsche Price (3)` - Go to RStudio Cloud and open `Porsche Price (3)` --- class: middle # in·fer·ence a conclusion reached on the basis of evidence and reasoning. --- # Inference * so far we've only been able to make claims about our sample -- * for example, we've just been describing `\(\hat{\beta}_1\)`, the _estimated_ slope of the relationship between `\(x\)` and `\(y\)`. -- * what if we want to extend these claims to the population? --- # Sparrow data _So far, we've been looking at a sample of 116 sparrows from Kent Island._ 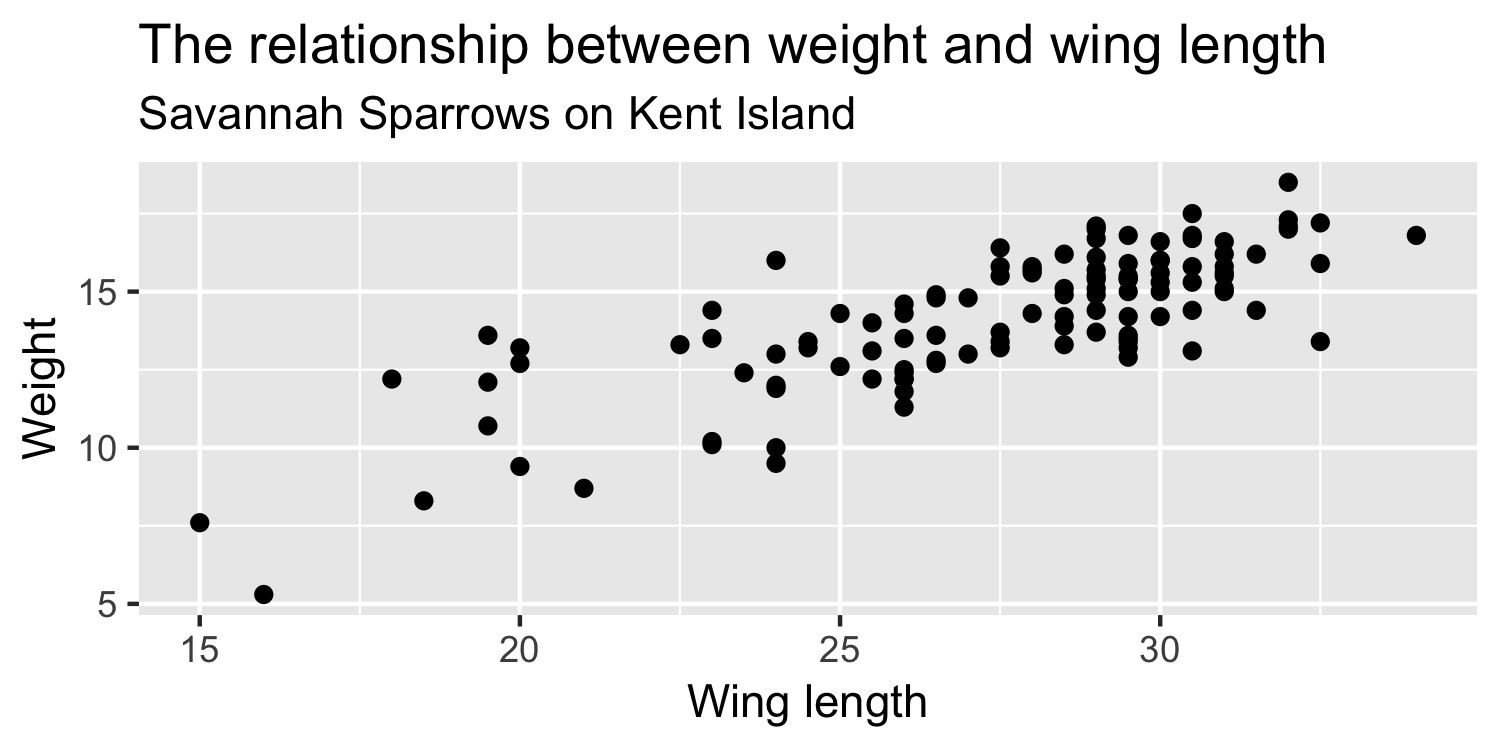<!-- --> --- ## Sparrows .question[ What if this were the true population, and the sample that we saw was just related _by chance_? ] 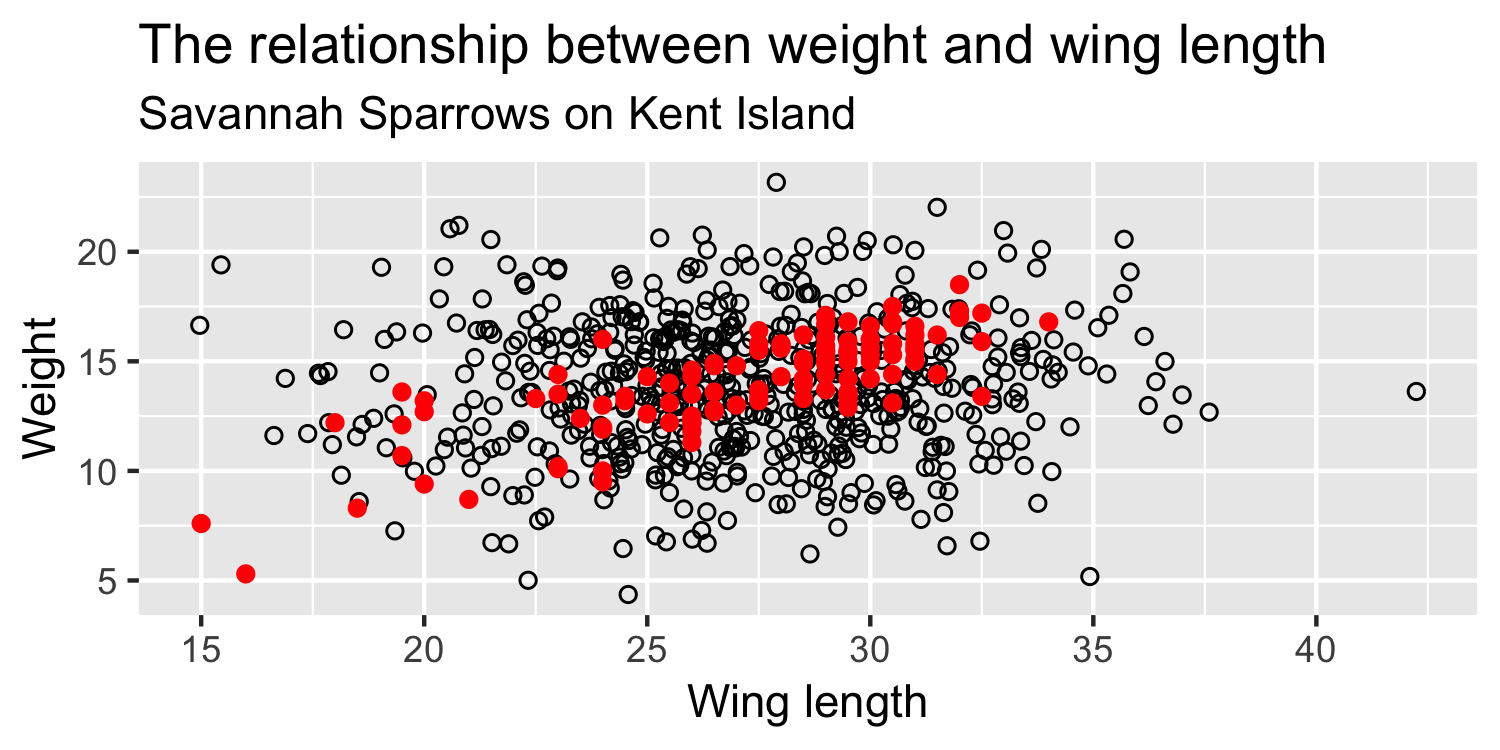<!-- --> --- ## Sparrows .question[ Ultimately What do we want to know? ] 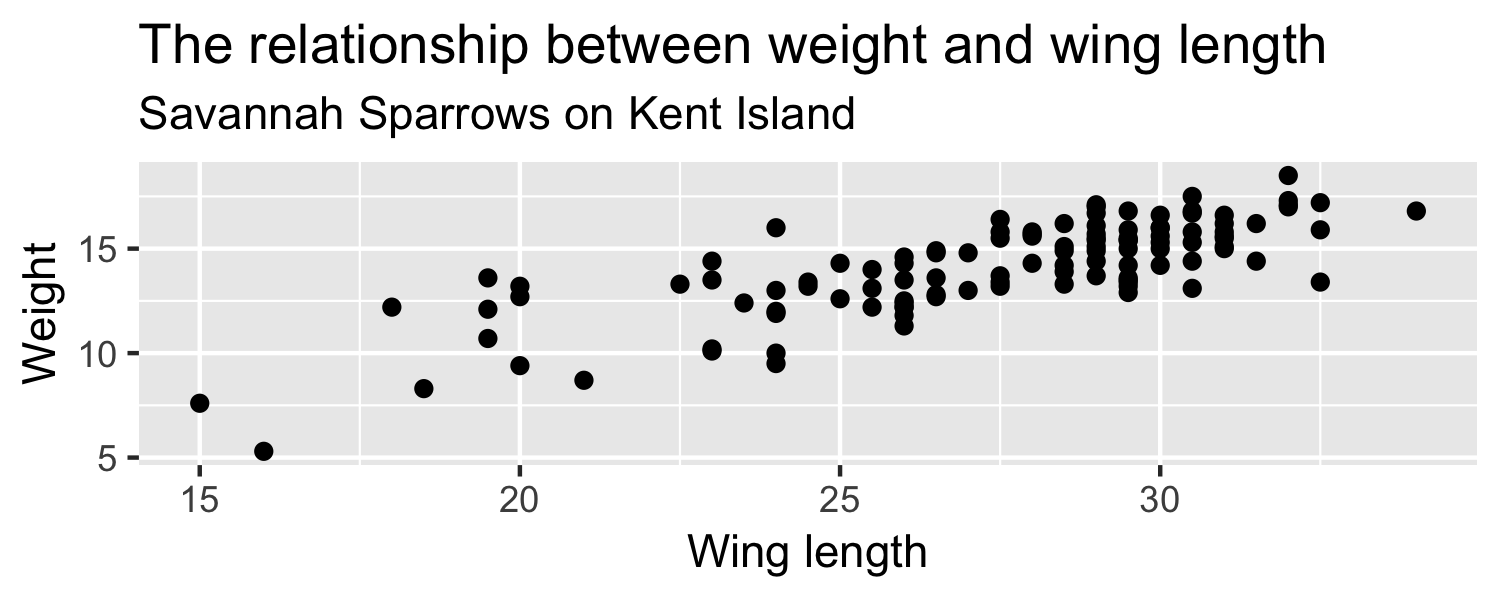<!-- --> --- ## Sparrows .question[ Ultimately What do we want to know? ] 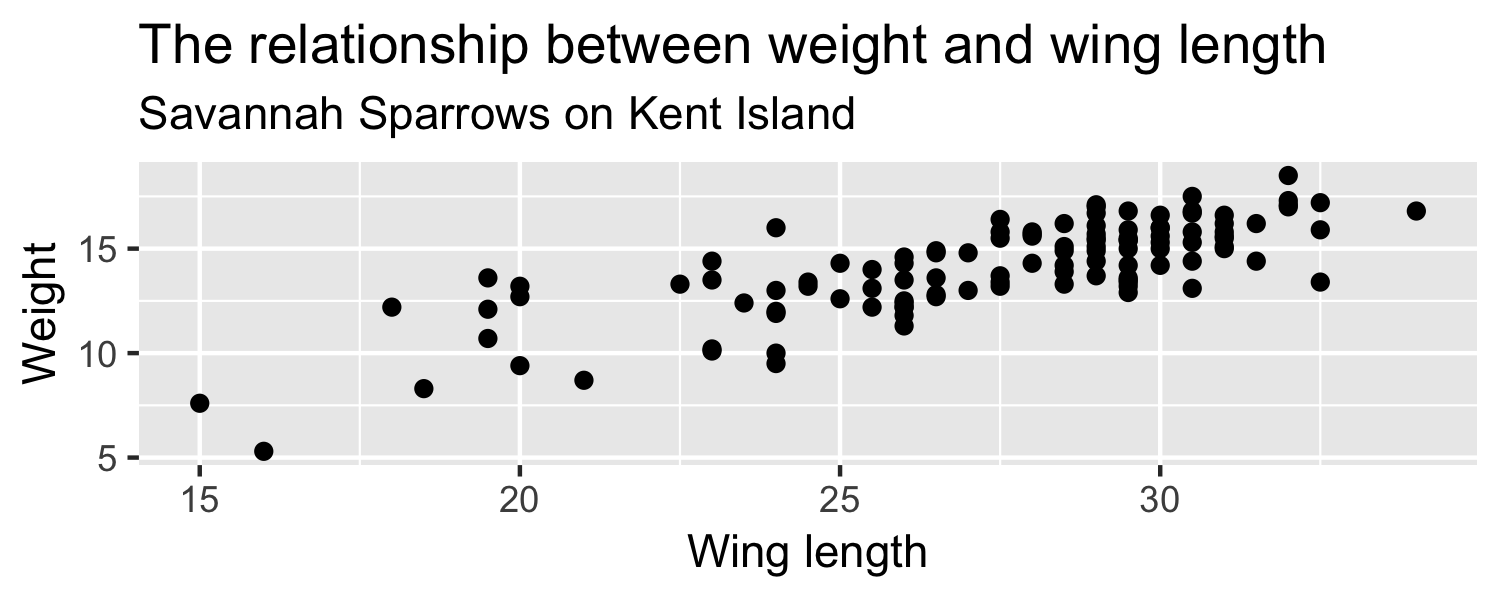<!-- --> * Does the slope in the **population** differ from 0? --- ## Sparrows .question[ Ultimately What do we want to know? ] 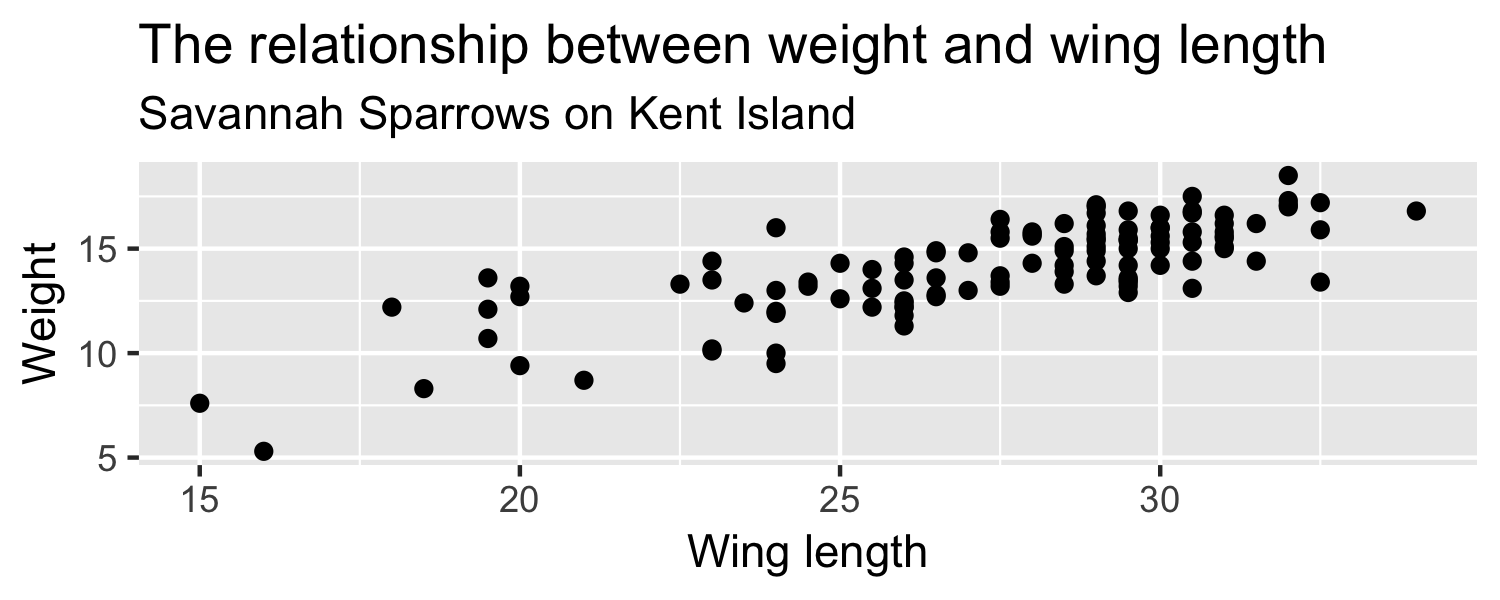<!-- --> * Does `\(\beta_1\)` differ from 0? -- * _notice the lack of a hat!_ --- ## Sparrows .question[ Ultimately What do we want to know? ] 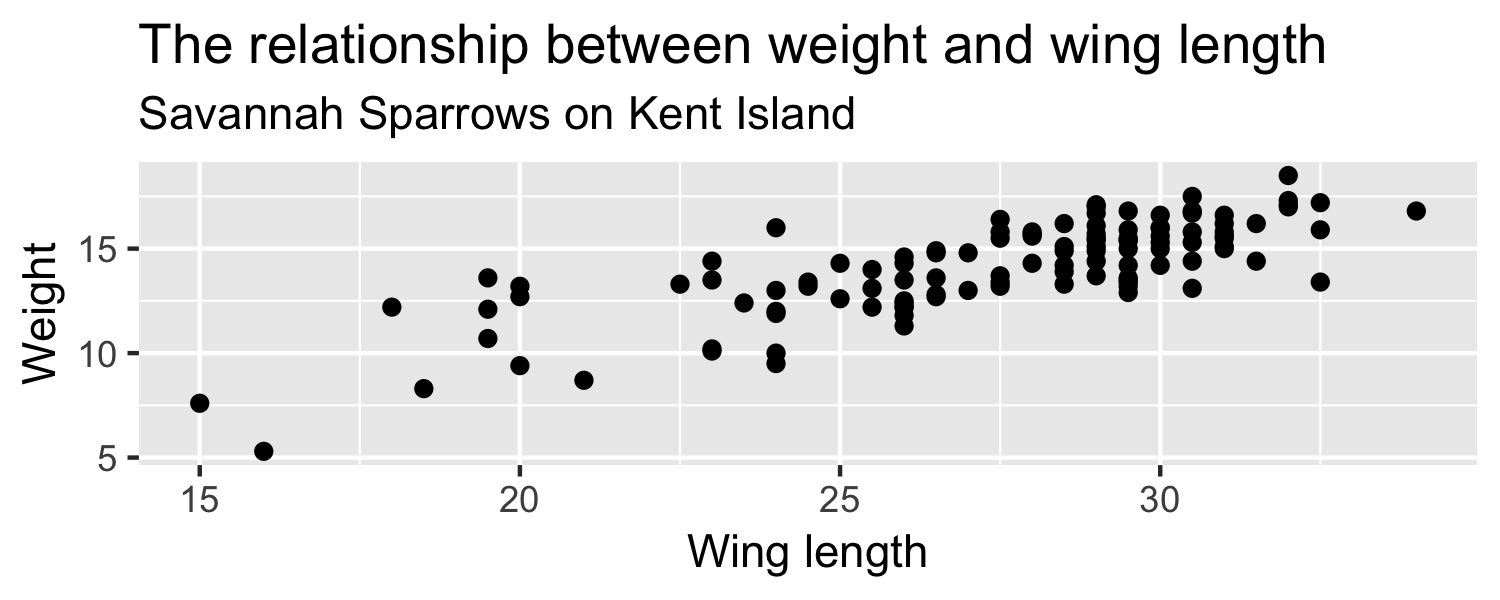<!-- --> * **null hypothesis** `\(H_0: \beta_1 = 0\)` * **alternative hypothesis** `\(H_A: \beta_1 \ne 0\)` --- ## Sparrows .question[ How can we quantify how much we'd expect the slope to differ from one random sample to another? ] 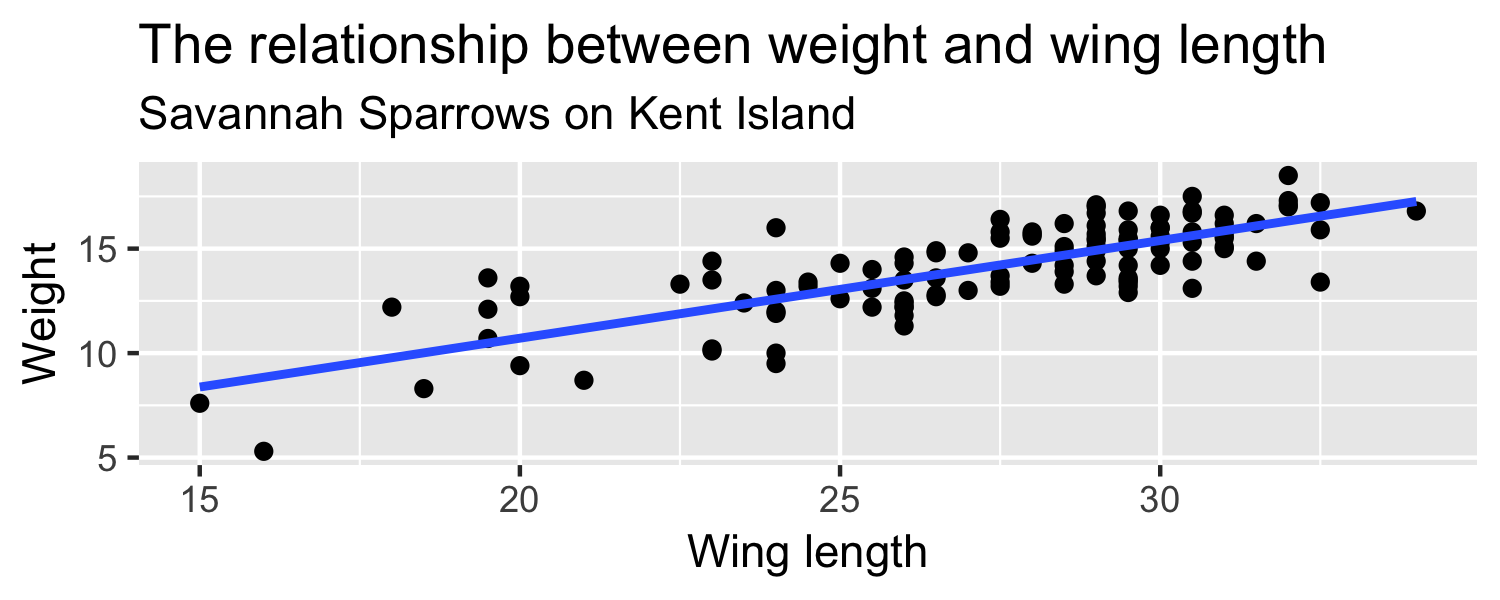<!-- --> --- ## Sparrows .question[ How can we quantify how much we'd expect the slope to differ from one random sample to another? ] 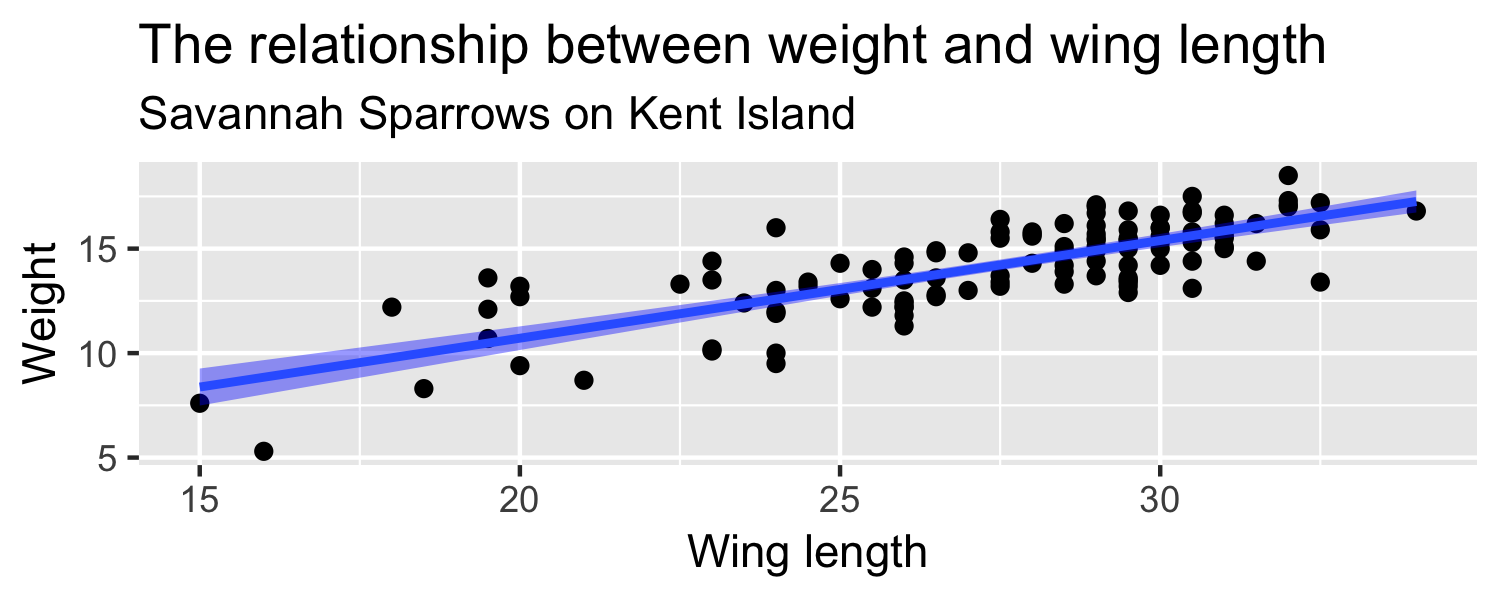<!-- --> * We need a measure of **uncertainty** --- ## Sparrows .question[ How can we quantify how much we'd expect the slope to differ from one random sample to another? ] 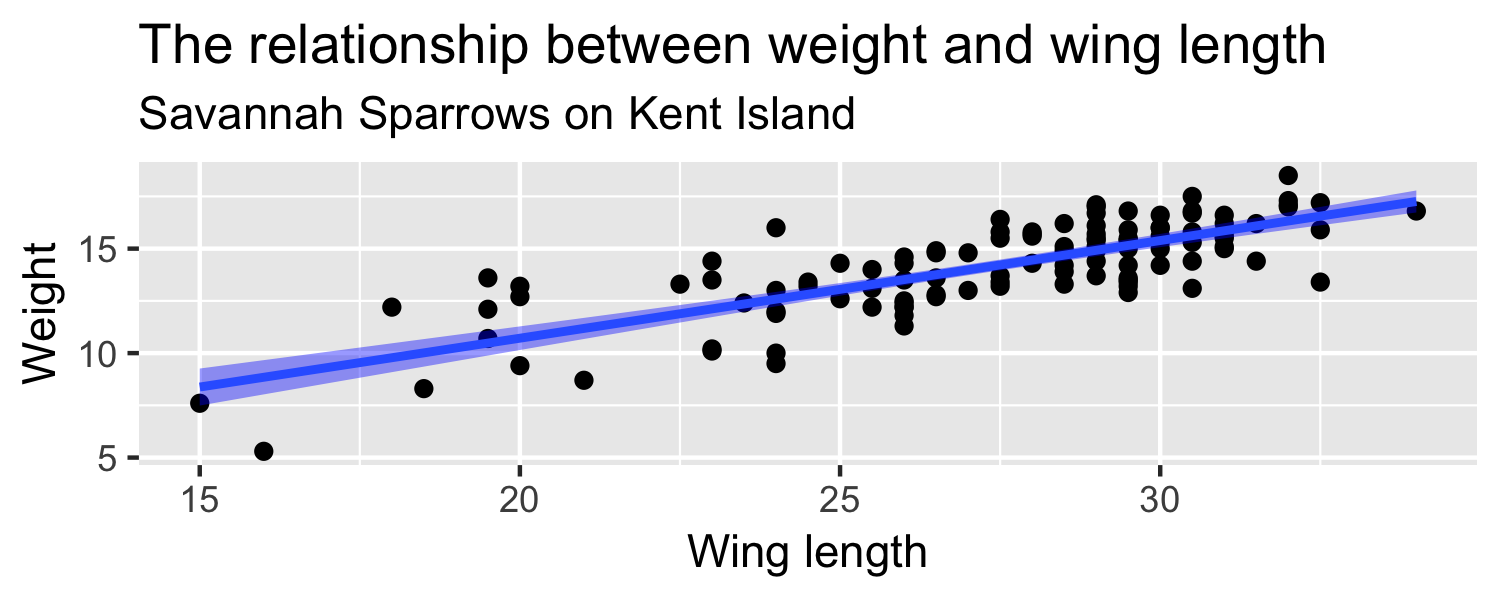<!-- --> * How about the **standard error** of `\(\hat{\beta}_1\)`? --- ## Sparrows .question[ How can we quantify how much we'd expect the slope to differ from one random sample to another? ] 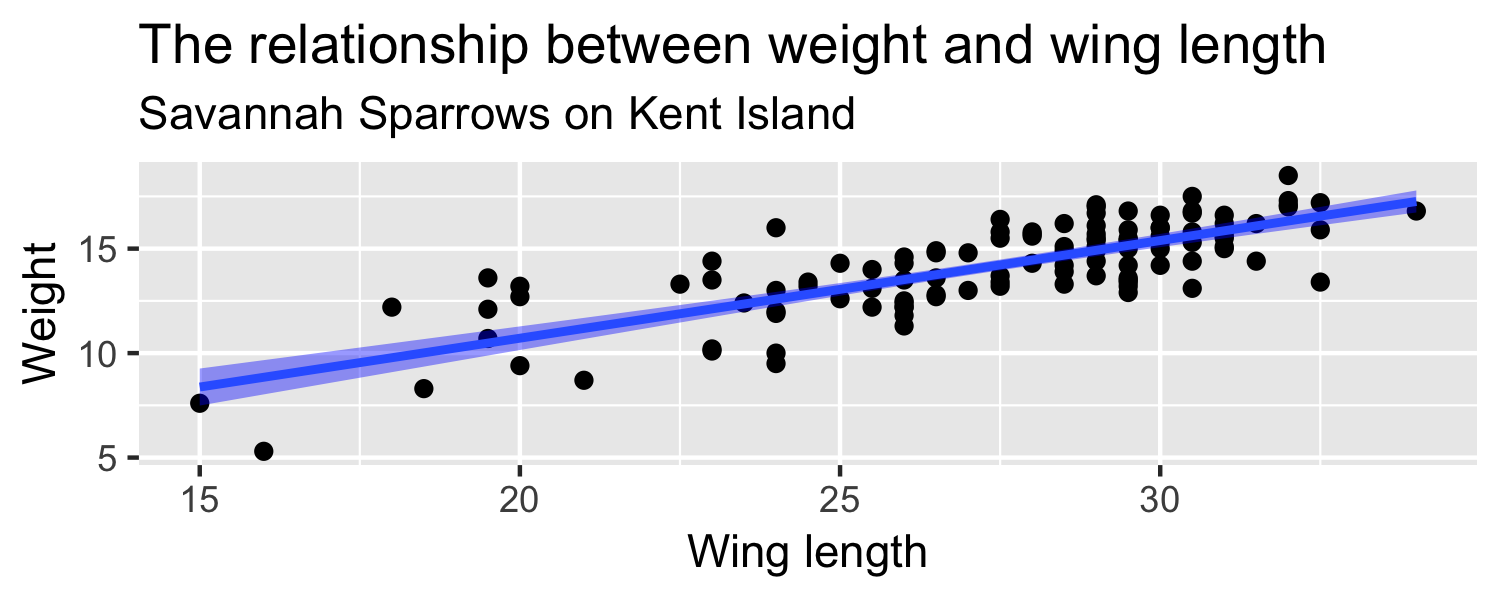<!-- --> * the **standard error** of `\(\hat{\beta_1}\)` ( `\(SE_{\hat{\beta}_1}\)` ) is how much we expect the sample slope to vary from one random sample to another. --- ## Sparrows .question[ How can we quantify how much we'd expect the slope to differ from one random sample to another? ] .small[ ```r lm(Weight ~ WingLength, data = Sparrows) %>% tidy() ``` ``` ## # A tibble: 2 x 5 ## term estimate std.error statistic p.value ## <chr> <dbl> <dbl> <dbl> <dbl> ## 1 (Intercept) 1.37 0.957 1.43 1.56e- 1 *## 2 WingLength 0.467 0.0347 13.5 2.62e-25 ``` ] --- ## Sparrows .question[ We need a **test statistic** that incorporates `\(\hat{\beta}_1\)` and the standard error `\(SE_{\hat\beta_1}\)` ] .small[ ```r lm(Weight ~ WingLength, data = Sparrows) %>% tidy() ``` ``` ## # A tibble: 2 x 5 ## term estimate std.error statistic p.value ## <chr> <dbl> <dbl> <dbl> <dbl> ## 1 (Intercept) 1.37 0.957 1.43 1.56e- 1 *## 2 WingLength 0.467 0.0347 13.5 2.62e-25 ``` ] --- ## Sparrows .question[ We need a **test statistic** that incorporates `\(\hat{\beta}_1\)` and the standard error `\(SE_{\hat\beta_1}\)` ] .small[ ```r lm(Weight ~ WingLength, data = Sparrows) %>% tidy() ``` ``` ## # A tibble: 2 x 5 ## term estimate std.error statistic p.value ## <chr> <dbl> <dbl> <dbl> <dbl> ## 1 (Intercept) 1.37 0.957 1.43 1.56e- 1 *## 2 WingLength 0.467 0.0347 13.5 2.62e-25 ``` ] # `\(t = \frac{\hat\beta_1}{SE_{\hat\beta_1}}\)` --- ## Sparrows .question[ We need a **test statistic** that incorporates `\(\hat{\beta}_1\)` and the standard error `\(SE_{\hat\beta_1}\)` ] .small[ ```r lm(Weight ~ WingLength, data = Sparrows) %>% tidy() ``` ``` ## # A tibble: 2 x 5 ## term estimate std.error statistic p.value ## <chr> <dbl> <dbl> <dbl> <dbl> ## 1 (Intercept) 1.37 0.957 1.43 1.56e- 1 *## 2 WingLength 0.467 0.0347 13.5 2.62e-25 ``` ] ```r 0.467 / 0.0347 ``` ``` ## [1] 13.45821 ``` --- ## Sparrows .question[ How do we interpret this? ] .small[ ```r lm(Weight ~ WingLength, data = Sparrows) %>% tidy() ``` ``` ## # A tibble: 2 x 5 ## term estimate std.error statistic p.value ## <chr> <dbl> <dbl> <dbl> <dbl> ## 1 (Intercept) 1.37 0.957 1.43 1.56e- 1 *## 2 WingLength 0.467 0.0347 13.5 2.62e-25 ``` ] ```r 0.467 / 0.0347 ``` ``` ## [1] 13.45821 ``` --- ## Sparrows .question[ How do we interpret this? ] .small[ ```r lm(Weight ~ WingLength, data = Sparrows) %>% tidy() ``` ``` ## # A tibble: 2 x 5 ## term estimate std.error statistic p.value ## <chr> <dbl> <dbl> <dbl> <dbl> ## 1 (Intercept) 1.37 0.957 1.43 1.56e- 1 *## 2 WingLength 0.467 0.0347 13.5 2.62e-25 ``` ] * "the sample slope is more than 13 standard errors above a slope of zero" --- ## Sparrows .question[ How do we know what values of this statistic are worth paying attention to? ] .small[ ```r lm(Weight ~ WingLength, data = Sparrows) %>% tidy() ``` ``` ## # A tibble: 2 x 5 ## term estimate std.error statistic p.value ## <chr> <dbl> <dbl> <dbl> <dbl> ## 1 (Intercept) 1.37 0.957 1.43 1.56e- 1 *## 2 WingLength 0.467 0.0347 13.5 2.62e-25 ``` ] --- ## Sparrows .question[ How do we know what values of this statistic are worth paying attention to? ] .small[ ```r lm(Weight ~ WingLength, data = Sparrows) %>% tidy() ``` ``` ## # A tibble: 2 x 5 ## term estimate std.error statistic p.value ## <chr> <dbl> <dbl> <dbl> <dbl> ## 1 (Intercept) 1.37 0.957 1.43 1.56e- 1 *## 2 WingLength 0.467 0.0347 13.5 2.62e-25 ``` ] * confidence intervals * p-values --- ## Sparrows .question[ How do we know what values of this statistic are worth paying attention to? ] .small[ ```r lm(Weight ~ WingLength, data = Sparrows) %>% * tidy(conf.int = TRUE) ``` ``` ## # A tibble: 2 x 7 ## term estimate std.error statistic p.value conf.low conf.high ## <chr> <dbl> <dbl> <dbl> <dbl> <dbl> <dbl> ## 1 (Intercept) 1.37 0.957 1.43 1.56e- 1 -0.531 3.26 *## 2 WingLength 0.467 0.0347 13.5 2.62e-25 0.399 0.536 ``` ] * confidence intervals * p-values --- ## Sparrows .question[ Where do these come from? ] .small[ ```r lm(Weight ~ WingLength, data = Sparrows) %>% tidy(conf.int = TRUE) ``` ``` ## # A tibble: 2 x 7 ## term estimate std.error statistic p.value conf.low conf.high ## <chr> <dbl> <dbl> <dbl> <dbl> <dbl> <dbl> ## 1 (Intercept) 1.37 0.957 1.43 1.56e- 1 -0.531 3.26 *## 2 WingLength 0.467 0.0347 13.5 2.62e-25 0.399 0.536 ``` ] * confidence intervals * p-values --- ## Sparrows .question[ What if we knew what the distribution of the "statistic" would be under the null hypothesis? ] .small[ ```r lm(Weight ~ WingLength, data = Sparrows) %>% tidy() ``` ``` ## # A tibble: 2 x 5 ## term estimate std.error statistic p.value ## <chr> <dbl> <dbl> <dbl> <dbl> ## 1 (Intercept) 1.37 0.957 1.43 1.56e- 1 *## 2 WingLength 0.467 0.0347 13.5 2.62e-25 ``` ] --- ## Sparrows ```r null_sparrow_data <- data.frame( WingLength = rnorm(10, 27, 4), Weight = rnorm(10, 14, 3) ) lm(Weight ~ WingLength, data = null_sparrow_data) %>% tidy() ``` ``` ## # A tibble: 2 x 5 ## term estimate std.error statistic p.value ## <chr> <dbl> <dbl> <dbl> <dbl> ## 1 (Intercept) 6.17 3.55 1.74 0.121 ## 2 WingLength 0.334 0.129 2.58 0.0326 ``` --- ## Sparrows ```r null_sparrow_data <- data.frame( * WingLength = rnorm(10, 27, 4), Weight = rnorm(10, 14, 3) ) lm(Weight ~ WingLength, data = null_sparrow_data) %>% tidy() ``` ``` ## # A tibble: 2 x 5 ## term estimate std.error statistic p.value ## <chr> <dbl> <dbl> <dbl> <dbl> ## 1 (Intercept) 11.2 5.96 1.88 0.0968 ## 2 WingLength 0.0840 0.206 0.407 0.695 ``` --- ## Sparrows ```r null_sparrow_data <- data.frame( WingLength = rnorm(10, 27, 4), * Weight = rnorm(10, 14, 3) ) lm(Weight ~ WingLength, data = null_sparrow_data) %>% tidy() ``` ``` ## # A tibble: 2 x 5 ## term estimate std.error statistic p.value ## <chr> <dbl> <dbl> <dbl> <dbl> ## 1 (Intercept) 11.7 5.94 1.97 0.0846 ## 2 WingLength 0.121 0.223 0.543 0.602 ``` --- ## Sparrows .small[ ```r *gen_null_stat <- function() { null_sparrow_data <- data.frame( WingLength = rnorm(10, 27, 4), Weight = rnorm(10, 14, 3) ) lm(Weight ~ WingLength, data = null_sparrow_data) %>% tidy() %>% filter(term == "WingLength") %>% select("statistic") *} ``` ] ```r gen_null_stat() ``` ``` ## # A tibble: 1 x 1 ## statistic ## <dbl> ## 1 -0.661 ``` --- ## Sparrows .small[ ```r gen_null_stat() ``` ``` ## # A tibble: 1 x 1 ## statistic ## <dbl> ## 1 -0.422 ``` ```r gen_null_stat() ``` ``` ## # A tibble: 1 x 1 ## statistic ## <dbl> ## 1 0.536 ``` ```r gen_null_stat() ``` ``` ## # A tibble: 1 x 1 ## statistic ## <dbl> ## 1 -2.19 ``` ] --- ## Sparrows ```r null_stats <- map_df(1:10000, ~ gen_null_stat()) ``` --- ## Sparrows ```r null_stats <- map_df(1:10000, ~ gen_null_stat()) ``` 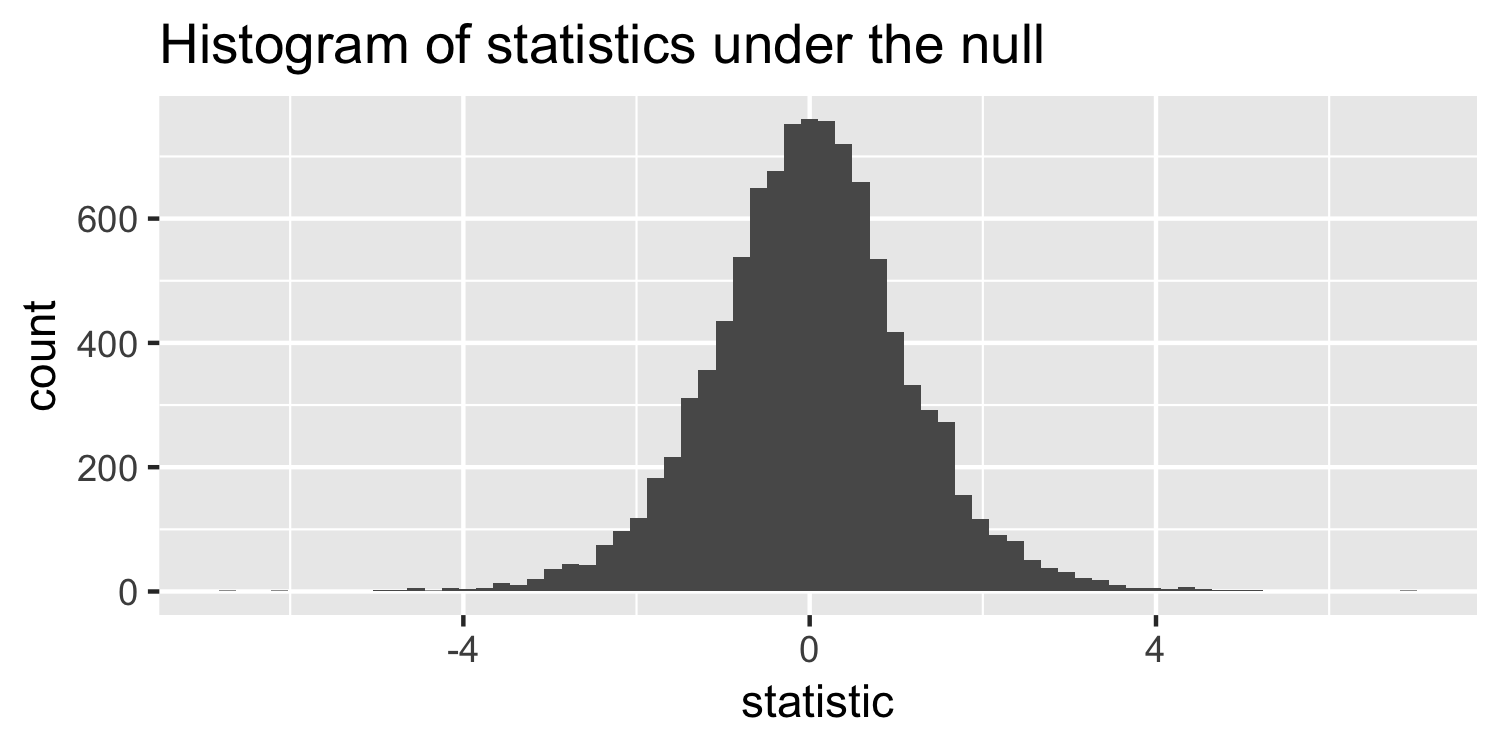<!-- --> --- ## Sparrows .question[ What distribution does this look like? ] 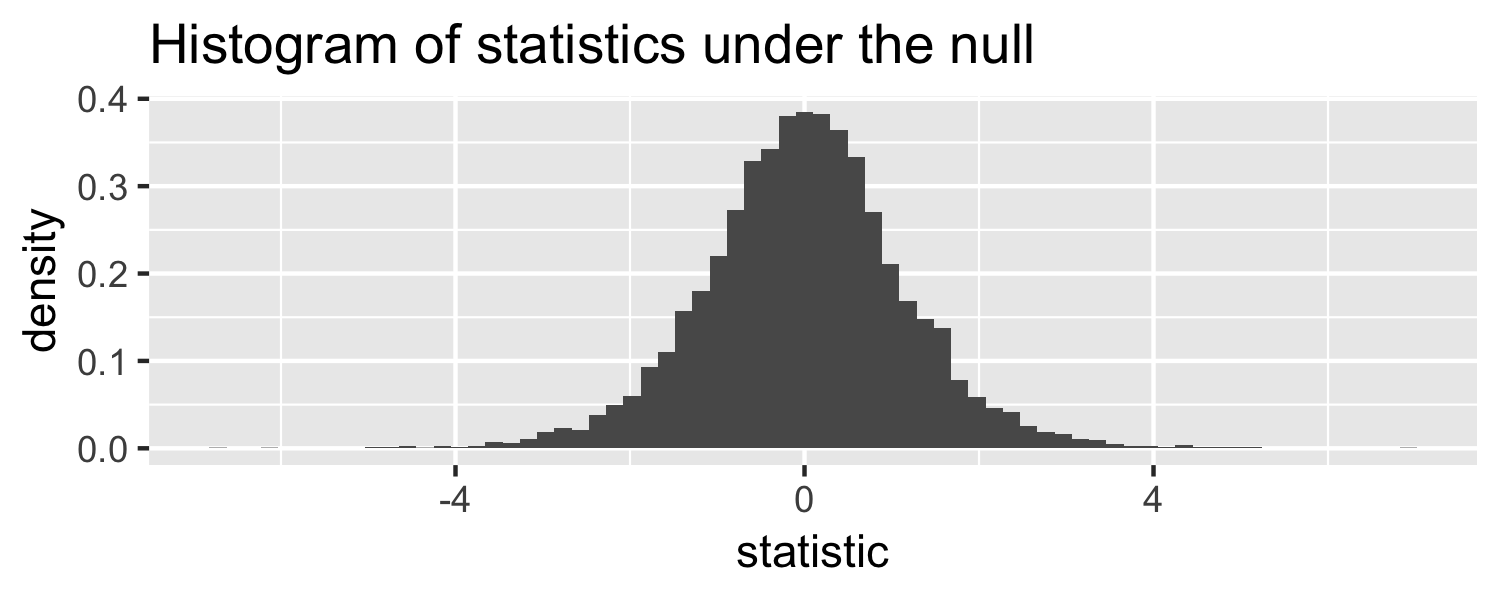<!-- --> --- ## Sparrows .question[ What distribution does this look like? ] 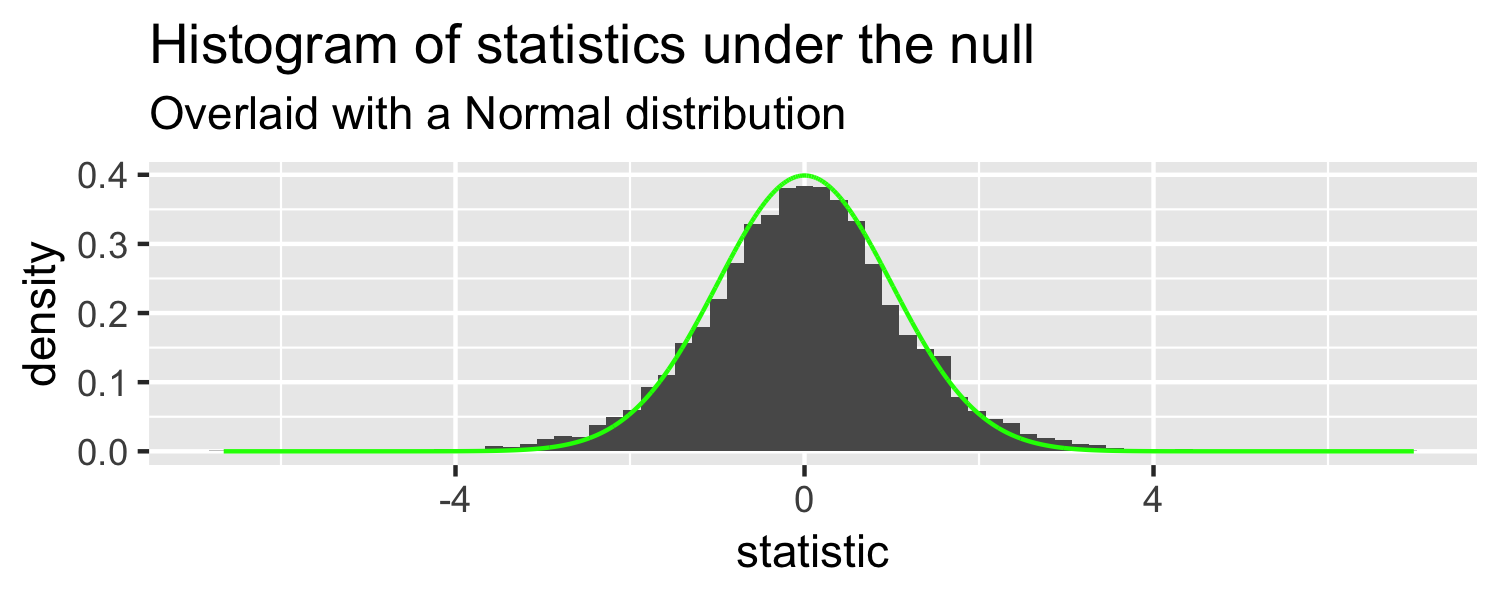<!-- --> * Normal? -- * What distribution is similar to the normal but with fatter tails? --- ## Sparrows .question[ What distribution does this look like? ] 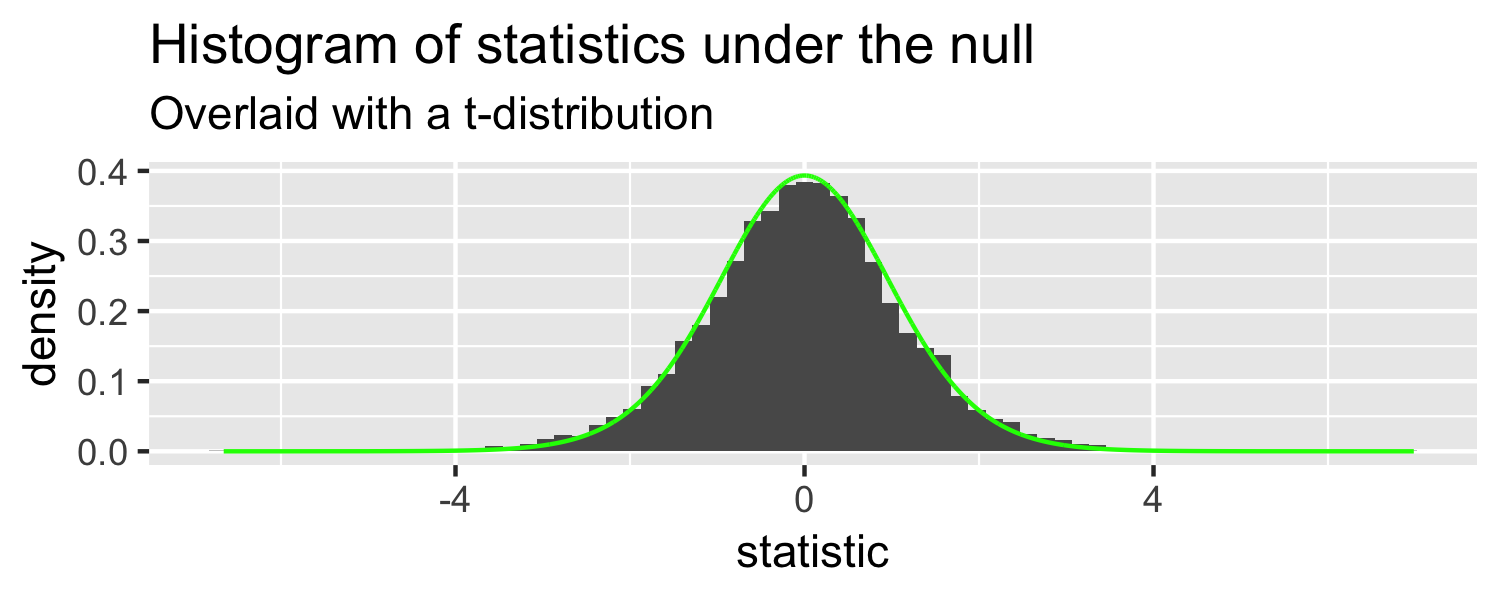<!-- --> * the *t-distribution!* -- * this is a **t-distribution** with **n-2** degrees of freedom. --- ## Sparrows The distribution of test statistics we would expect given the **null hypothesis is true**, `\(\beta_1 = 0\)`, is **t-distribution** with **n-2** degrees of freedom. 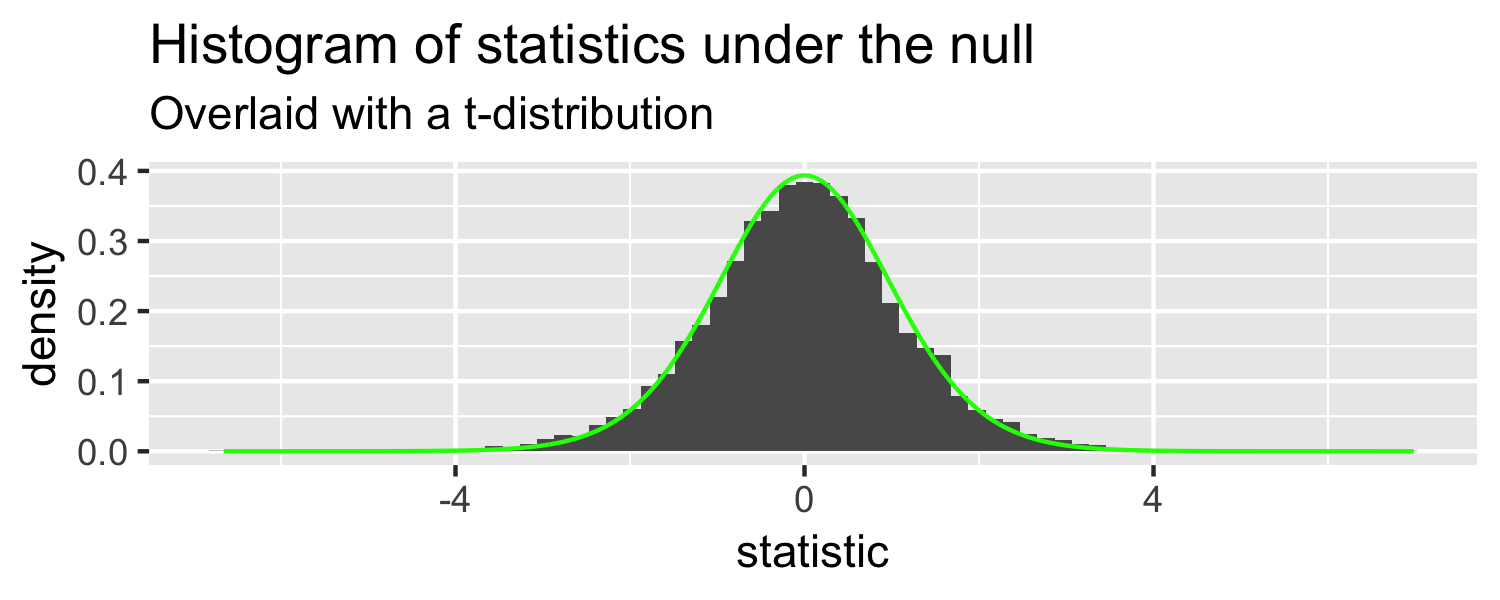<!-- --> --- ## Sparrows 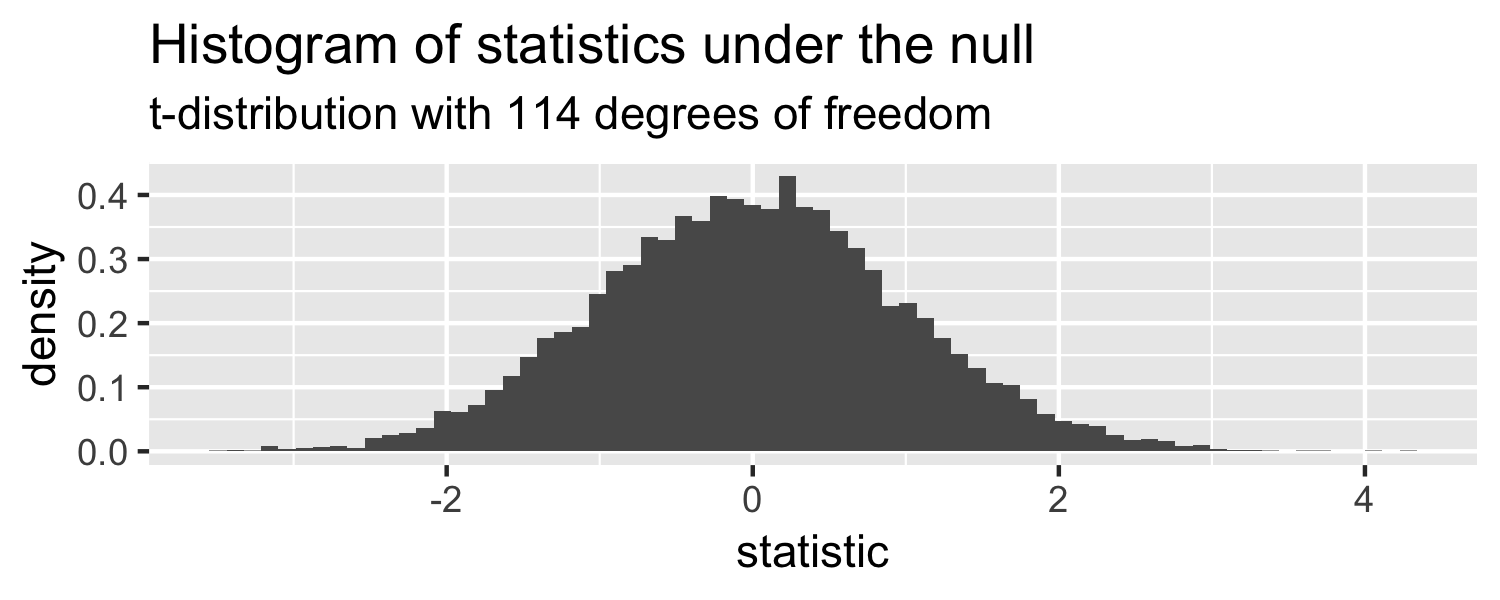<!-- --> .small[ ```r lm(Weight ~ WingLength, data = Sparrows) %>% tidy() ``` ``` ## # A tibble: 2 x 5 ## term estimate std.error statistic p.value ## <chr> <dbl> <dbl> <dbl> <dbl> ## 1 (Intercept) 1.37 0.957 1.43 1.56e- 1 *## 2 WingLength 0.467 0.0347 13.5 2.62e-25 ``` ] --- ## Sparrows 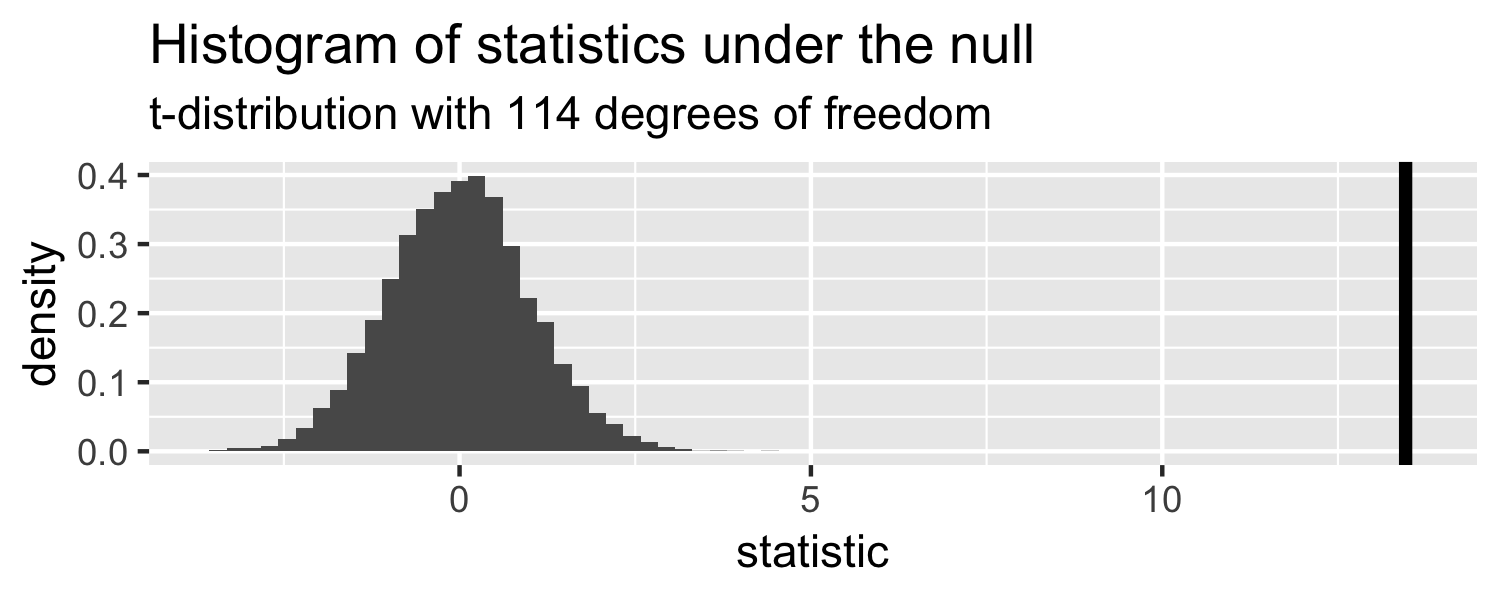<!-- --> --- ## Sparrows .question[ How can we compare this line to the distribution under the null? ] 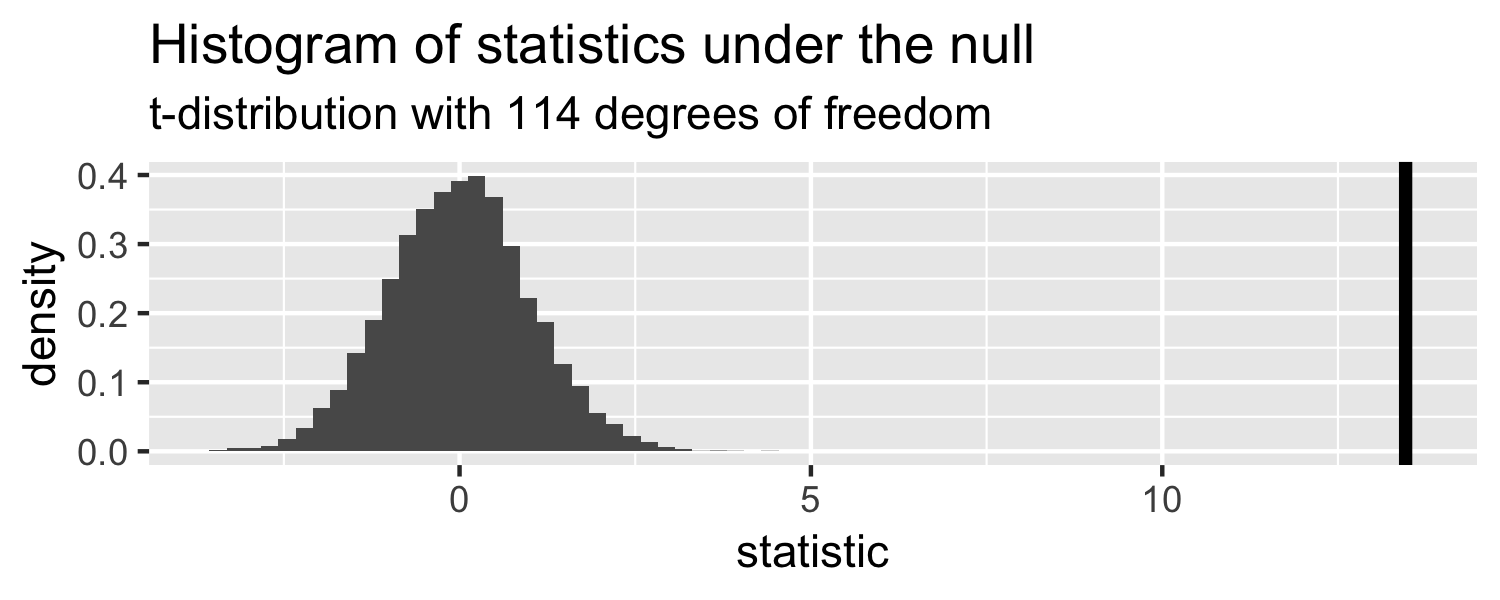<!-- --> -- * p-value --- class: middle # p-value The probability of getting a statistic as extreme or more extreme than the observed test statistic **given the null hypothesis is true** --- ## Sparrows 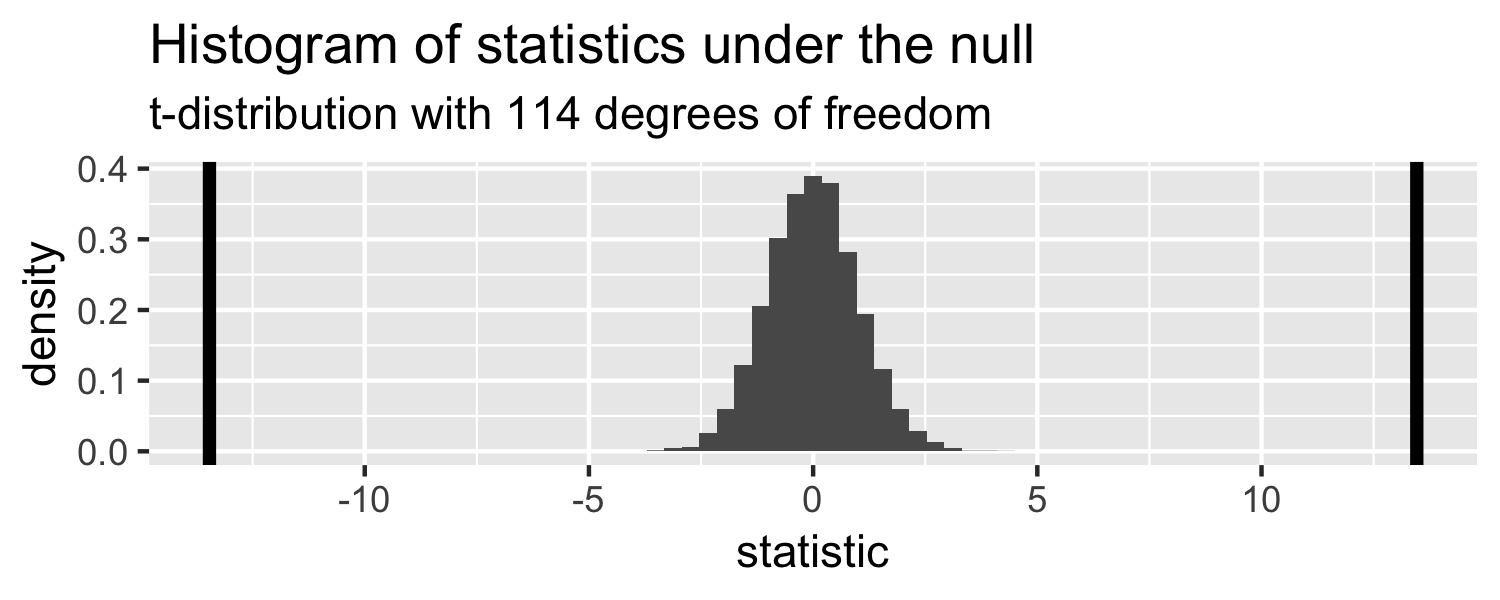<!-- --> .small[ ```r lm(Weight ~ WingLength, data = Sparrows) %>% tidy() ``` ``` ## # A tibble: 2 x 5 ## term estimate std.error statistic p.value ## <chr> <dbl> <dbl> <dbl> <dbl> ## 1 (Intercept) 1.37 0.957 1.43 1.56e- 1 *## 2 WingLength 0.467 0.0347 13.5 2.62e-25 ``` ] --- ## Return to generated data, n = 20 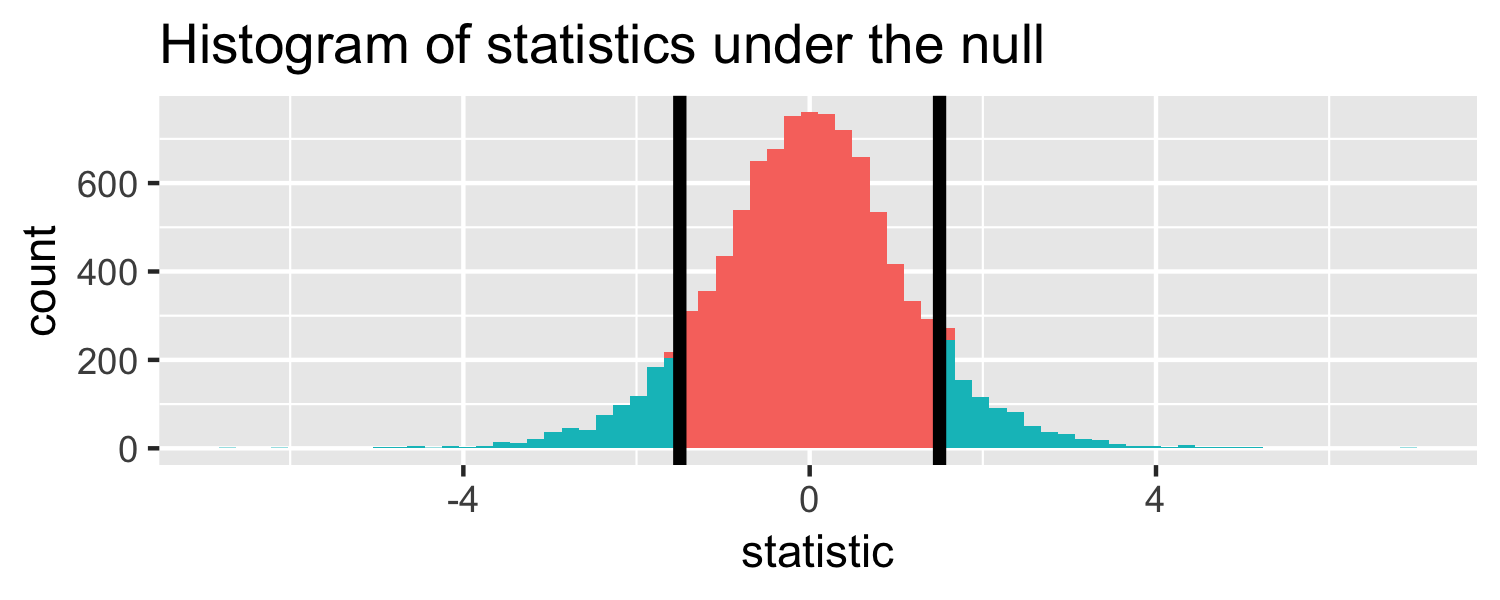<!-- --> * Let's say we get a statistic of **1.5** in a sample --- ## Let's do it in R! The proportion of area less than 1.5 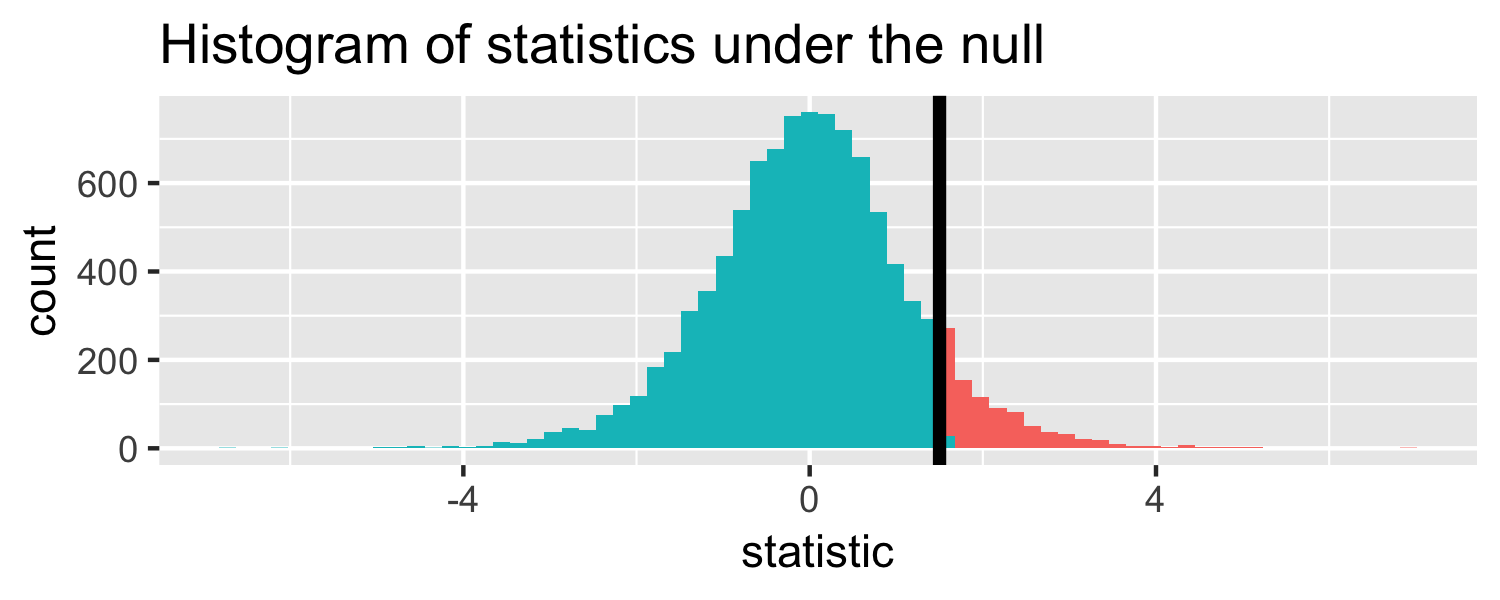<!-- --> ```r pt(1.5, df = 18) ``` ``` ## [1] 0.9245248 ``` --- ## Let's do it in R! The proportion of area **greater** than 1.5 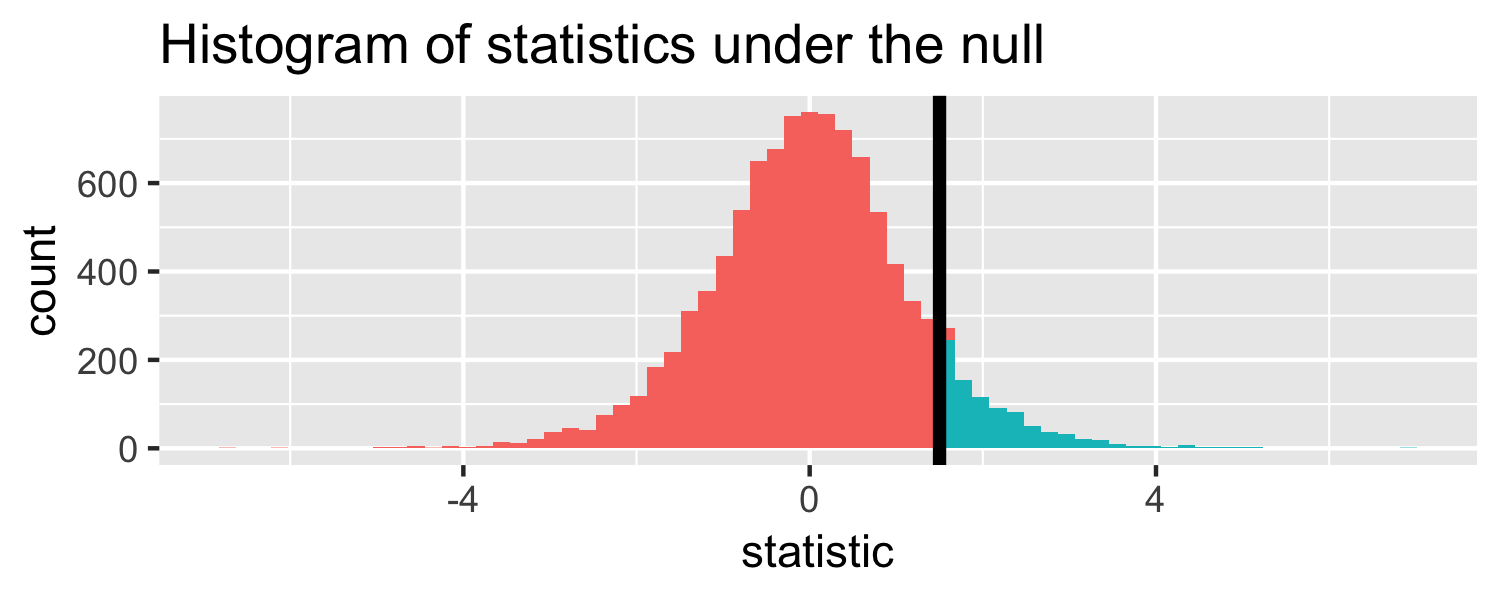<!-- --> ```r pt(1.5, df = 18, lower.tail = FALSE) ``` ``` ## [1] 0.07547523 ``` --- ## Let's do it in R! The proportion of area **greater** than 1.5 **or** **less** than -1.5. 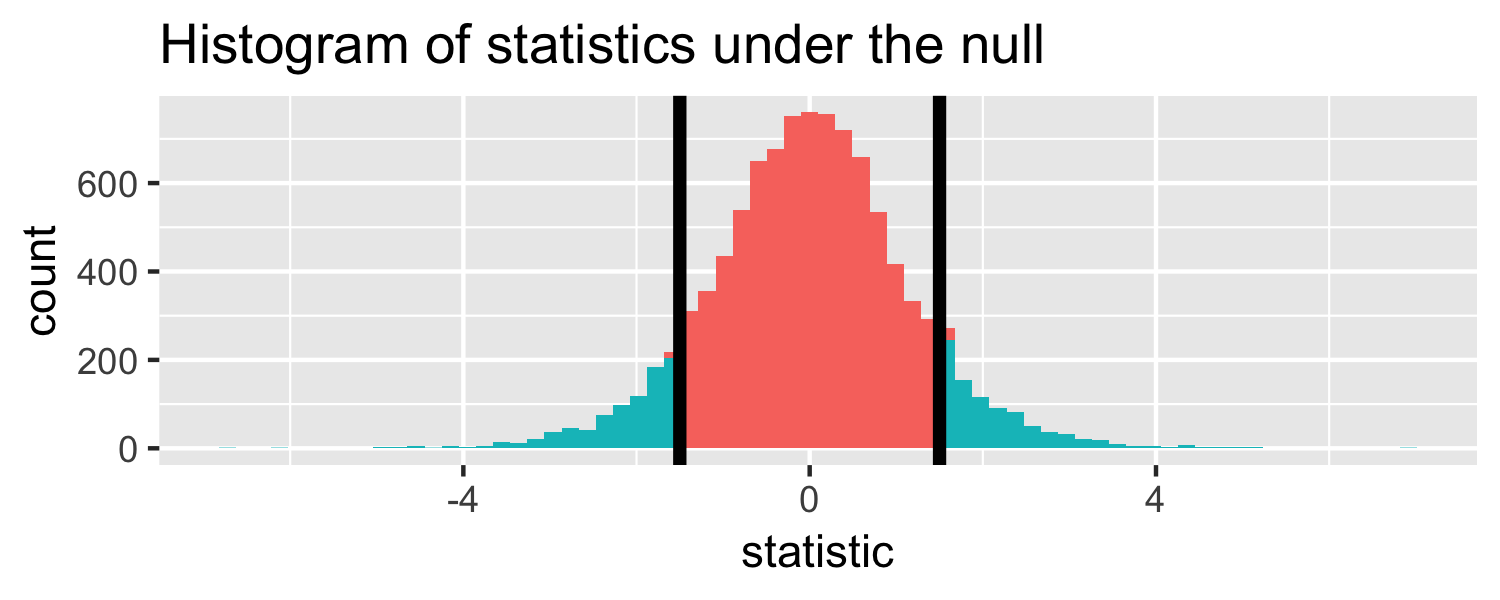<!-- --> -- ```r pt(1.5, df = 18, lower.tail = FALSE) * 2 ``` ``` ## [1] 0.1509505 ``` --- class: middle # p-value The probability of getting a statistic as extreme or more extreme than the observed test statistic **given the null hypothesis is true** --- ## Hypothesis test * **null hypothesis** `\(H_0: \beta_1 = 0\)` * **alternative hypothesis** `\(H_A: \beta_1 \ne 0\)` -- * **p-value**: 0.15 -- * Often, we have an `\(\alpha\)`-level cutoff to compare this to, for example **0.05**. Since this is greater than **0.05**, we **fail to reject the null hypothesis** --- class: middle # confidence intervals If we use the same sampling method to select different samples and computed an interval estimate for each sample, we would expect the true population parameter ( `\(\beta_1\)` ) to fall within the interval estimates 95% of the time. --- # Confidence interval .center[ `\(\Huge \hat\beta_1 \pm t^∗ \times SE_{\hat\beta_1}\)` ] -- * `\(t^*\)` is the critical value for the `\(t_{n−2}\)` density curve to obtain the desired confidence level -- * Often we want a **95% confidence level**. --- ## Let's do it in R! .small[ ```r lm(Weight ~ WingLength, data = Sparrows) %>% tidy(conf.int = TRUE) ``` ``` ## # A tibble: 2 x 7 ## term estimate std.error statistic p.value conf.low conf.high ## <chr> <dbl> <dbl> <dbl> <dbl> <dbl> <dbl> ## 1 (Intercept) 1.37 0.957 1.43 1.56e- 1 -0.531 3.26 *## 2 WingLength 0.467 0.0347 13.5 2.62e-25 0.399 0.536 ``` ] ```r qt(0.025, df = nrow(Sparrows) - 2, lower.tail = FALSE) ``` ``` ## [1] 1.980992 ``` --- ## Let's do it in R! .question[ Why 0.025? ] .small[ ```r lm(Weight ~ WingLength, data = Sparrows) %>% tidy(conf.int = TRUE) ``` ``` ## # A tibble: 2 x 7 ## term estimate std.error statistic p.value conf.low conf.high ## <chr> <dbl> <dbl> <dbl> <dbl> <dbl> <dbl> ## 1 (Intercept) 1.37 0.957 1.43 1.56e- 1 -0.531 3.26 *## 2 WingLength 0.467 0.0347 13.5 2.62e-25 0.399 0.536 ``` ] ```r qt(0.025, df = nrow(Sparrows) - 2, lower.tail = FALSE) ``` ``` ## [1] 1.980992 ``` --- ## Let's do it in R! .question[ Why `lower.tail = FALSE`? ] .small[ ```r lm(Weight ~ WingLength, data = Sparrows) %>% tidy(conf.int = TRUE) ``` ``` ## # A tibble: 2 x 7 ## term estimate std.error statistic p.value conf.low conf.high ## <chr> <dbl> <dbl> <dbl> <dbl> <dbl> <dbl> ## 1 (Intercept) 1.37 0.957 1.43 1.56e- 1 -0.531 3.26 *## 2 WingLength 0.467 0.0347 13.5 2.62e-25 0.399 0.536 ``` ] ```r qt(0.025, df = nrow(Sparrows) - 2, lower.tail = FALSE) ``` ``` ## [1] 1.980992 ``` --- ## Let's do it in R! .small[ ```r lm(Weight ~ WingLength, data = Sparrows) %>% tidy(conf.int = TRUE) ``` ``` ## # A tibble: 2 x 7 ## term estimate std.error statistic p.value conf.low conf.high ## <chr> <dbl> <dbl> <dbl> <dbl> <dbl> <dbl> ## 1 (Intercept) 1.37 0.957 1.43 1.56e- 1 -0.531 3.26 *## 2 WingLength 0.467 0.0347 13.5 2.62e-25 0.399 0.536 ``` ] ```r t_star <- qt(0.025, df = nrow(Sparrows) - 2, lower.tail = FALSE) 0.467 + t_star * 0.0347 ``` ``` ## [1] 0.536 ``` ```r 0.467 - t_star * 0.0347 ``` ``` ## [1] 0.398 ``` --- class: middle # confidence intervals If we use the same sampling method to select different samples and computed an interval estimate for each sample, we would expect the true population parameter ( `\(\beta_1\)` ) to fall within the interval estimates 95% of the time. --- ## <i class="fas fa-laptop"></i> `Porsche Price (3)` - Go to RStudio Cloud and open `Porsche Price (3)`